Welcome to HBH! If you have tried to register and didn't get a verification email, please using the following link to resend the verification email.
Artificial Intelligence - C Code Bank
Artificial Intelligence
This code implements a multilayer neural network for computing functions. It is currently set up to compute 2-bit XOR but could easily be modified to do other interesting things. Currently I am coding a newer version for handwriting recognition and satellite image classification for my A.I. class.
/* A back-propagation learning multilayer neural network
*
* Parameterized to allow for easy modification of the number of hidden and input neurons
* which will adjust the number of weights automatically. Hard-coded for a two layer network.
* This will work for most problems; any continuous function may be represented.
* An additional layer allows for even discontinuous functions to be represented.
*
*/
#include <stdio.h>
#include <stdlib.h>
#include <string.h>
#include <unistd.h>
#include <math.h>
#include <time.h>
#include <sys/types.h>
#include <sys/stat.h>
#include <fcntl.h>
#include <arpa/inet.h>
#define BIAS -1.0 /* activation value of the bias neuron */
#define NUM_I 2 /* number of input neurons - w/o bias */
#define NUM_H 3 /* number of hidden neurons - w/o bias */
#define NUM_O 1 /* number of output neurons */
#define NPATTS 4 /* number of patterns in the training set */
#define EPOCHS 1000 /* number of times to cycle through the example set */
int **getIntPatts(void), xor(int), trainNet(int **, double *, double *);
int BackProp(int, int, double *, double *);
double **initWeights(int, int), sigmoid(double), sigmoidPrime(double);
void FeedForward(int, double *, double *);
const float lrate = 1.0;
double *sumsh,*sumso; // stores the weighted sums for each layer of neurons
double **wih, **who; // stores the weights for each layer
int main(int argc, char **argv)
{
int **patterns = NULL;
double *netout = malloc(sizeof(double) * NUM_O); // what the network thinks
double *hidout = malloc(sizeof(double) * NUM_H); // results from hidden layer
sumsh = malloc(sizeof(double) * NUM_H); // weighted summations for hidden layer
sumso = malloc(sizeof(double) * NUM_O); // weighted summations for output layer
srand(time(NULL)*getpid());
patterns = getIntPatts(); // generate some patterns for xor or whatever
/* initialize the weights; small, random values */
wih = initWeights(NUM_I, NUM_H); // weights between input and hidden layer
who = initWeights(NUM_H, NUM_O); // weights between hidden and output layer
/* train this thing. */
printf(\"%s\\n\", trainNet(patterns, netout, hidout) ? \"Epoch limit exceeded!\" : \"Should be ready.\");
free(sumsh); free(sumso); free(patterns); free(wih); free(who);
return 0;
}
int trainNet(int **p, double *netout, double *hidout)
{
int i,j;
for (i=0; i<EPOCHS; i++) {
for (j=0; j<NPATTS; j++) {
FeedForward(p[j][0], hidout, netout);
BackProp(p[j][0], p[j][1], hidout, netout);
}
}
free(netout); free(hidout);
return 1;
}
/*
* Take the input pattern one bit at a time and propagate forward to calculate output.
* Compute the weighted sum for each layer, if we are using the bias weight then use
* -1.0 for the activation value from the previous layer.
*/
void FeedForward(int pattern, double *hidout, double *netout)
{
int i,j;
// Compute activations over input layer for the hidden neurons
memset(sumsh, 0, sizeof(double) * NUM_H);
for (i=0; i<NUM_H; i++) {
for (j=0; j<NUM_I+1; j++)
sumsh[i] += (j>=NUM_I ? (BIAS*wih[j][i]) : (((1 << j) & pattern ? 1.0 : 0.0)*wih[j][i]));
hidout[i] = sigmoid(sumsh[i]);
}
// Compute activations over hidden layer for output neurons
memset(sumso, 0, sizeof(double) * NUM_O);
for (i=0; i<NUM_O; i++) {
for (j=0; j<NUM_H+1; j++)
sumso[i] += (j>=NUM_H ? BIAS*who[j][i] : hidout[j]*who[j][i]);
netout[i] = sigmoid(sumso[i]);
}
}
/*
*
* Back Propagation algorithm:
* the output given by the network after forward propagation of the input is compared
* with the true value for the input to compute some measure of error. any amount of error
* will be potentially caused by any layer in the network, thus the error value will be
* propagated backward through the layers and used to update the weights of those layers\'
* weights.
*
*/
int BackProp(int pattern, int target, double *hidout, double *netout)
{
int i,j,k;
double *err = malloc(sizeof(double) * NUM_O); // stores the errors for the output layer
double *deltao = malloc(sizeof(double) * NUM_O);// stores the deltas for the output layer
double *deltah = malloc(sizeof(double) * NUM_H);// stores the deltas for the hidden layer
memset(deltao, 0, sizeof(double) * NUM_O);
memset(deltah, 0, sizeof(double) * NUM_H);
// Compute hidden to output layer deltas
for (i=0; i<NUM_O; i++) {
err[i] = target - netout[i];
printf(\"%d, %d - %f = err=%f\\n\",pattern,target,netout[i],err[i]);
deltao[i] = err[i] * sigmoidPrime(sumso[i]);
}
// Compute input to hidden layer deltas
for (i=0; i<NUM_H; i++) {
for (j=0; j<NUM_O; j++)
deltah[i] += sigmoidPrime(sumsh[i])*who[i][j]*deltao[j];
}
// Update who
for (i=0; i<NUM_O; i++) {
for (j=0; j<NUM_H+1; j++)
who[j][i] += (j>=NUM_H ? lrate*BIAS*deltao[i] : lrate*hidout[j]*deltao[i]);
}
// Update wih
for (j=0; j<NUM_H; j++) {
for (k=0; k<NUM_I+1; k++)
wih[k][j] += (k>=NUM_I ? lrate*BIAS*deltah[j] : lrate*((1 << k) & pattern ? 1 : 0)*deltah[j]);
}
// Clean up
free(err); free(deltao); free(deltah);
return 1;
}
double sigmoid(double x)
{
return 1.0/(1.0 + exp(-1.0*x));
}
double sigmoidPrime(double x)
{
return sigmoid(x)*(1 - sigmoid(x));
}
/*
* create an array of patterns along with their true values
* this function is useful for simple functions
*/
int **getIntPatts()
{
// however many patterns we specify
int **patts = malloc(sizeof(int *) * NPATTS),i;
for (i=0; i<NPATTS; i++) {
// space enough for two integers
patts[i] = malloc(sizeof(int) * 2);
// first int is random number with number of bits equal to number of input neurons
//patts[i][0] = random() % (1 << NUM_I);
// second int is the xor of the bits from the first int
//patts[i][1] = xor(patts[i][0]) ? 1 : 0;
}
// For 2-bit XOR it\'s easy enough just to hardcode all possible values.
patts[0][0] = 0;
patts[0][1] = 0;
patts[1][0] = 1;
patts[1][1] = 1;
patts[2][0] = 2;
patts[2][1] = 1;
patts[3][0] = 3;
patts[3][1] = 0;
return patts;
}
/* Compute XOR of int x */
int xor(int x)
{
/* XOR on more than 2 bits means an odd number of 1s */
int i,ones=0,zeros=0;
for (i=0; i<NUM_I; i++)
x & (1 << i) ? ones++ : zeros++;
return (ones % 2) ? 1 : 0;
}
/*
* Initialize weights to small, random values between -1.0 and 1.0.
* - organized into \"groups\" by feeding layer, n1
*/
double **initWeights(int n1, int n2)
{
int i,j;
double **weights = malloc(sizeof(double *) * (n1+1)); // include an extra for the bias neuron
for (i=0; i<n1+1; i++) {
weights[i] = malloc(sizeof(double) * n2);
// this is my way of getting a random number between -1.0 and 1.0.
for (j=0; j<n2; j++)
weights[i][j] = (random() % 2 ? (double)random() : -1.0*(double)random())/(double)RAND_MAX;
}
return weights;
}
Comments
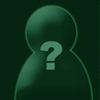